Image recognition (IR) tech has been around for some time, and when it was first introduced it sounded promising. From simplifying stock counts to streamlining in-store audits, image recognition set out to be an essential tool for optimising retail execution and enhancing the customer experience. However, there remain certain drawbacks to the use of IR as we know it. For instance, exactly how it is trained has a ripple effect in terms of its effectiveness, and how reliable it really is are critical concerns that retailers must navigate. Perhaps for these reasons, according, a recent POI report, only 54% of CPG’s are currently utilising image recognition.Computer vision is essentially the “brain” of image recognition, so the data used to train the technology is vital. Generating real data (i.e. photos and manual annotations) to train traditional IR technologies, especially in retail contexts, is expensive, time-consuming to collect and not scalable. Therefore, brand and field marketing agencies are increasingly turning to synthetic computer vision (SCV) to aid in this process. SCV uses computer-generated digital twins of SKUs and retail settings to train its image recognition algorithms. It represents the next evolution of IR technology.
The use of SCV and synthetic data stands to transform how CPG brands, Field Marketing Agencies (FMA) and Sales Force Automation (SFA) companies use and deploy image recognition tech. So, are you ready to try a better approach to IR? Read on to learn about the advantages of switching your image recognition from real data to synthetic data.

The Five Advantages of Synthetic Data in Image Recognition
Synthetic data refers to computer-generated data that is used to train machine learning models for image recognition tasks. This data can be generated programmatically and can be used to create a wide variety of images, including objects and scenes, without the need for expensive and time-consuming data collection processes. By using synthetic data, you can unlock new potential within your image recognition, including:
1. Greater accuracy
Synthetic data provides greater accuracy than real data, and results are more reliable. Synthetic data also reduces the restrictions on what you can do with your IR tech. For instance, when connected to an SFA solution, synthetic IR can improve planogram compliance, shelf compliance, product availability, price analysis, competitor analysis and promotional display compliance to name a few. Synthetic data offers a distinct advantage over real data when it comes to training algorithms. Generating vast volumes of diverse data with synthetic data creates a more robust training data set, which helps to ensure that product detection accuracy does not degrade over time. This also allows algorithms to handle even the most challenging store environments, with difficult lighting conditions or deformations of product packaging.
2. Low complexity to set up and use the technology
Gathering real data to train IR algorithms is not scalable, because it is costly, time-consuming and labour-intensive. By contrast, synthetic image recognition creates hyper-realistic 3D digital twin of your products and begins training the algorithm in a matter of minutes.
This revolutionary approach to image recognition is transforming the industry and our first of a kind technology, ZIA (Zero Image Annotations) is training SCV algorithms to detect products from just their packaging label. This reduces the complexity of setting up and using the technology, as the 3D digital twin and the virtual scenes of the shop floor becomes the source of all your training data, meaning you don’t have to take pictures of a physical object multiple times. Instead, the SCV model can detect the product in 360 degrees, removing the need for human input throughout the entire operation.
With real data, you can only generate reactionary methods of IR, meaning you can only begin training a traditional computer vision model once the product exists on shelves. As a result, product analysis is delayed. However, synthetic IR allows you to expedite the process and generate an analysis before a product has even launched, simply by using the packaging label/artwork file.
3. Robust performance across different store situations and environments
Environmental factors like lighting, product distortions and even messy surroundings can alter the performance of traditional IR models that rely on real data. This is because they’re often trained on sterile marketing photos that assume ideal conditions. So, traditional IR models can struggle to accurately detect a product when it is on a busy shelf, deformed in some way or has less than ideal lighting compared to when it is by itself or alongside the same SKU.
By using synthetic data to build virtual scenes, computer vision algorithms can detect SKUs in a multitude of different environments, and within minutes. In turn, products are detected even in more challenging environmental conditions (which is typically the norm for real-world stores). Detecting deformities allows for more accurate results as products might be damaged by consumers or during transit, and traditional IR will likely not be able to detect the product as a result.
Alternatively, synthetic computer vision solutions can mimic both ideal and imperfect lighting situations as well as other sources of error, like product damage and mess. For example, ZIA has a robust performance track record that can detect products even if they are in a different state than when they were first uploaded onto our system.In practice, this means that our model is trained to detect a product that has been crushed, crumpled or deformed in a variety of ways. Best of all, since a digital twin isn’t real, the product can be deformed endless times without creating any waste. This means it’s good for the environment and your bottom line.
4. Faster product onboarding
Traditional image recognition technology using real data needs a considerable amount of imagery and time to be trained before it can be used. However, synthetic data allows for faster onboarding as the algorithm programmatically generates training data from just the product artwork/label. As an example, with ZIA, a customer can be onboarded up to four times faster than the market average. ZIA also has a database of over 100,000 existing SKUs that the SCV algorithm has already trained with that you can leverage from day one.
This speed enables CPGs to onboard a high volume of SKUs in a matter of hours and days rather than weeks or months. In turn, FMCG companies can speed up their market launch, ensure perfect store execution and get ahead.
5. Lower cost of set up and maintenance
Finally, synthetic image recognition technology is more cost efficient and faster to use than real data image recognition since you need to collect and label large datasets manually.
Additionally, synthetic data can be generated at scale, allowing for more extensive and varied datasets - which would be impossible to achieve at scale with real data. Also, a unique and competitive feature of synthetic image recognition is that it allows SKUs to be pre-loaded, meaning that new packaging and seasonal promotions can be detected as soon as they appear on the shelves, instead of having to react to them afterwards.
Access synthetic data solutions, and more, from Neurolabs
Interested in learning more about the potential of synthetic data for your retail execution? Request a demo today or download our ebook, where we explore how Synthetic Computer Vision is changing the game when applied to retail shelf auditing!
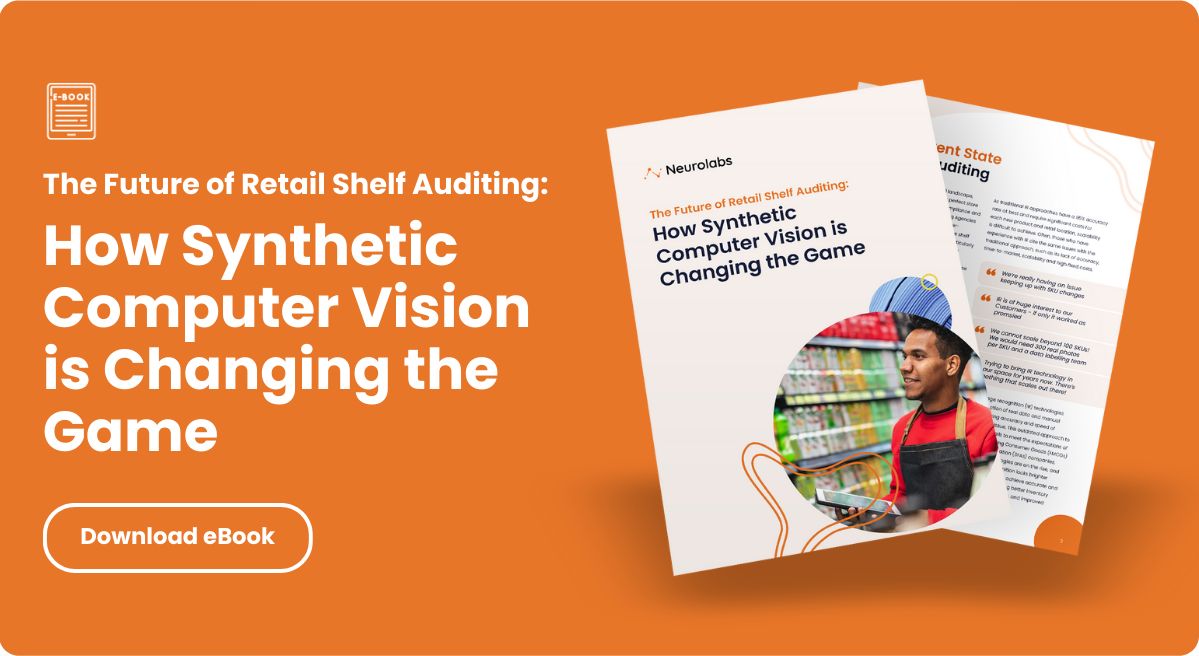
At Neurolabs, we are revolutionising in-store retail performance with our advanced image recognition technology, ZIA. Our cutting-edge technology enables retailers, field marketing agencies and CPG brands to optimise store execution, enhance the customer experience, and boost revenue as we build the most comprehensive 3D asset library for product recognition in the CPG industry.