At Neurolabs, we’re creating the technology that enables us to train Computer Vision algorithms by programmatically simulating realistic deformations in a wide range of packaging and materials. In this article, I'll discuss how this cutting-edge technology works when applied to identifying Consumer Packaged Goods (CPGs).
- What Are Deformations?
- What Problems Can Deformations Cause?
- The Benefits of Synthetic Data
- Pioneering Automated Deformation
- Faster and More Efficient Shelf Audits
What Are Deformations?
Packing for CPGs can be broadly classified into two categories: soft and rigid.
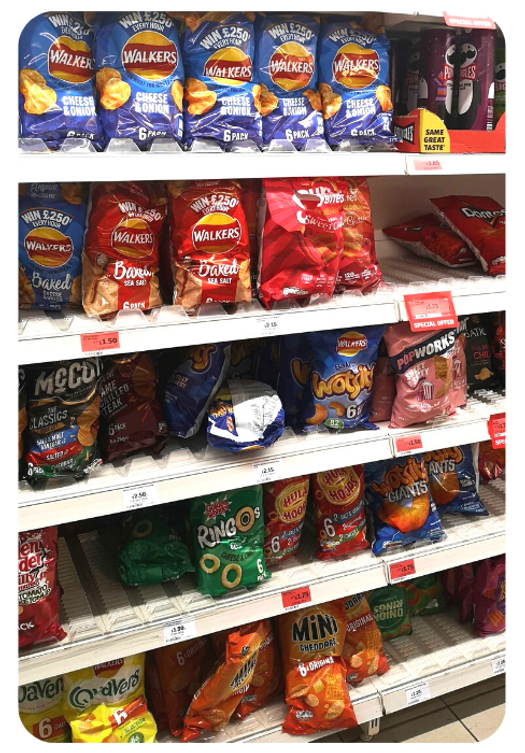
Deformations typically happen in the former and are essentially changes in the shape of soft body objects.
Soft body objects include packaging such as stand-up pouches with a ziplock, vacuum pouches (such as crisp packets), and laminated tubes (such as a tube of hand moisturiser).
These are popular packaging materials because they can be modified or customised with ease and are typically manufactured at low costs. The downside, however, is that they offer minimal protection from deformations which has developed into a major issue when it comes to product detection in the CPG space.
Rigid packaging is not immune to deformations either. Packaging such as aerosol spray cans, beverage cans, and bottles can also be impacted by deformations such as denting. In this article, I will focus on deformations that can occur while a soft body SKU is on the shelf, hence we won’t be discussing rigid object deformations.
What Problems Can Deformations Cause?
For retailers, product packaging deformation causes problems with stock management technology and can prevent you from knowing what’s happening on the shelf.
With rigid objects, such as a box, the variations in which you can find the SKU are limited. It can rotate, making it a somewhat simpler task for the computer vision technology to recognise the correct SKU in its varying positions.
With soft body objects, computer vision technology finds this much more difficult. If I picked up a packet of crisps from the shelf, I could deform it in numerous more ways than a rigid SKU. This could be anything from wrinkling the packaging to setting it back in a position where the store light now reflects on the label, making it visually unrecognisable to the image recognition (IR) software.
When I place the packet back on the shelf, it may have a deformation that the computer vision technology had not encountered before, and it would then no longer recognise it as a unit of stock.
The traditional approach to solving this problem has relied on collecting large amounts of training data. Many images are taken of an SKU in various deformed states, annotated, and fed into the computer vision technology. This method uses a real data approach, and collecting and annotating such data is time consuming, costly and prone to human error.
Deformations are not a simple challenge to solve and while real data presents one possible solution, it is far from perfect, taking up a lot of time and often still delivering inaccurate results. The number of variations that can occur to soft body packing is simply so high that it would be almost impossible to photograph and annotate a product in all the possible variations.
When deformations occur in a variation for which no real-world data has been collected, computer vision technology struggles to recognise it.
The unrecognised CPG leads to inaccurate data being reported, resulting in stock management issues such as overstocking or understocking. More importantly, unreliable data erodes users’ trust in the technology which has been designed to ultimately help them improve their efficiency. In fact, it can become a burden that slows them down.
These data blunders are far from insignificant. According to BusinessWire, retailers lose a mind-blowing amount of revenue each year due to poor inventory management. The total loss from out-of-stocks reaches $634.1 billion each year, and from overstocks, it's $471.9 billion. Combined, that makes annual losses of over $1 trillion.
As such, it is only logical for Field Force Managers and technology vendors, such as ourselves, to strive for a better alternative by pushing the limits of what is achievable, especially with such high stakes.
The Benefits of Synthetic Data
There is little doubt that real data is proving to be highly limited in the solutions it can provide. This is why at Neurolabs we decided to pioneer the use of synthetic data for image recognition of CPGs.
Synthetic data can be used as a (better) alternative to real-world data as it mimics real-world patterns and offers a faster, more diverse and more accurate way to create a database of imagery of a deformed product.
Neurolabs’ process to deform virtual products can simulate the realistic deformation of an item and create thousands of variations of the deformity using synthetic computer vision.
Neurolabs’ IR system - known as ZIA (Zero Image Annotations) - is powered by a combination of synthetic data and modern computer vision, with the ability to circumnavigate the need for real data altogether. These systems turn SKUs into digital 3D models for IR algorithms to learn from and reference, eliminating the need for time-consuming data collection.
For example, below are programmatically generated synthetic images of a crisp packet. The underlying 3D model of the crisp bag has been deformed automatically to increase the variation during the synthetic image generation process.

When we are automating the deformed product variants programmatically to train our algorithm, one of our main challenges lies in trying to ensure that the programmatically generated images are as realistic as possible to ensure accuracy when identifying real-world products.
Our AI is trained with synthetic data and the multitude of programmatically generated images of deformations, so it needs to be as realistic as possible. This way, when a field rep is in the store and takes a photo, our technology is advanced and accurate enough to detect deformed products on the shelf.
We are adding the recognition of deformations to our repository and our synthetic data approach using 3D assets. Although it's still early days, and the field of geometric deformations is vast and challenging, preliminary results show this is a promising approach for increasing variation and the performance of computer vision models.
We are striving to solve the issues seen in the CPG space, and deformations are another step in our journey.
Pioneering Automated Deformation
Our product, Neurolabs’ ZIA (Zero Image Annotations), is leading the way in automated geometric deformation and revolutionising accurate product detection in retail.
ZIA is a pioneering technology that tackles the challenge of ensuring the products and deformations rendered by synthetic data are accurate to the laws of physics and that their materials react as they would in the real world.
Geometric deformation has been explored in different industries with incredible results. The level of detail that it's possible to emulate is staggering. For example, some of the papers presented in the YouTube channel, Two Minute Papers, demonstrate methods of simulation and the tearing of meat in an astonishingly life-like way.
As a technology, geometric deformation is being explored and put to numerous uses. However, Neurolabs is the only company looking at simulating deformities using synthetic computer vision and applying them within the CPG space.
Deployed in the real world, ZIA recognises products in scope with +95% accuracy, notably higher than traditional IR with this increasing to above 98% for specific categories.
The solution that ZIA delivers to users provides them with a service to upload imagery of SKUs and a complete computer vision model that detects products in the real world and presents users with a viewable 3D asset that reflects a real-world product. The software is easily integrated into existing solutions or apps that Field Marketing Agencies use, simply enhancing your existing toolkit.
Faster and More Efficient Shelf Audits
Ready to start implementing a faster and more efficient shelf auditing process? Get an exclusive preview of ZIA today!
Get In Touch and one of our team will guide you through ZIA and how it can seamlessly integrate into your current solution and reduce product detection errors in your shelf auditing.
At Neurolabs, we are revolutionising in-store retail performance with our advanced image recognition technology, ZIA. Our cutting-edge technology enables retailers, field marketing agencies and CPG brands to optimise store execution, enhance the customer experience, and boost revenue as we build the most comprehensive 3D asset library for product recognition in the CPG industry.