In this study, we'll explore Neurolabs' impressive progress in using synthetic data to train computer vision models, from our first Vision Transformer to our advanced Vision Transformer V2.
Harnessing the Power of Synthetic Data
Our innovation lies in generating synthetic data. We've developed a method to procedurally create dense retail environments, allowing extensive variations in shelf structures, product assortments, and room layouts. This enables us to produce complex, annotation-rich synthetic datasets for various computer vision tasks.
Training our state-of-the-art vision transformer model on these datasets shows that synthetic data can match the performance of models trained on larger, real-data-dominated mixed datasets.
Remarkably, in some environments, our synthetic-only model outperforms those trained on mixed data, showcasing the effectiveness of our approach.
Datasets
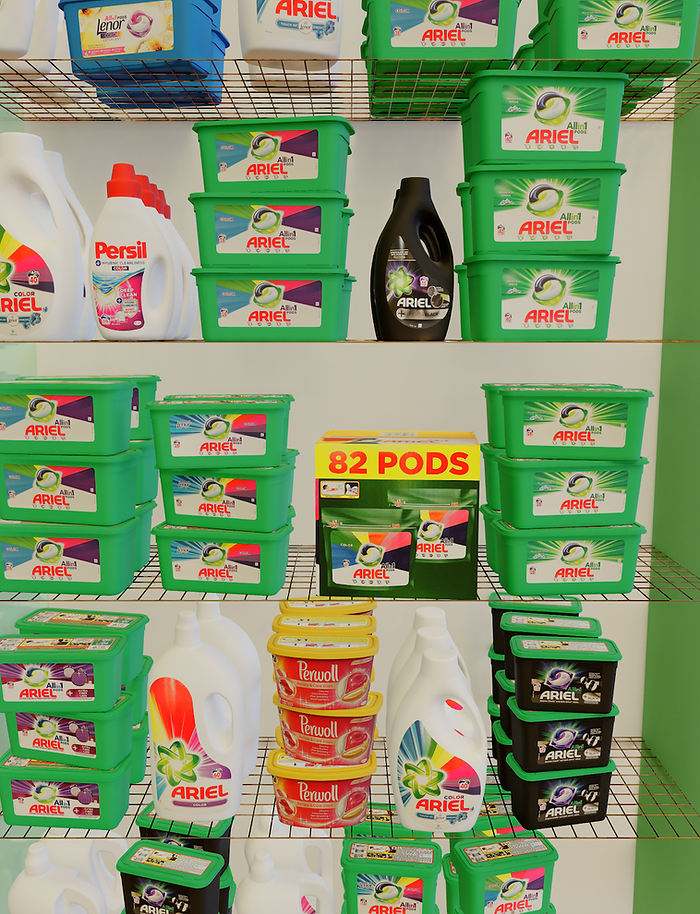
Our key datasets, NLB200k, Nuke1.0, and Nuke2.0, are crucial to enhancing model performance:
- NLB200k (Synthetic Only): Comprises procedurally generated retail environments with varied scene elements, allowing high performance using synthetic data alone, avoiding real data curation.
- Nuke1.0 and Nuke2.0 (Real + Synthetic): Feature real images supplemented with synthetic data for enhanced diversity and realism. They are designed for tasks like fine-grained classification and detailed scene understanding.
These meticulously crafted datasets ensure diversity and realism, enabling our models to excel in various retail scenarios.
Our Models
Our transition from Vision Transformer V1 to V2 marks a significant advancement. V2 processes more complex datasets, improving training times, performance, and additional features. Handling vast amounts of data efficiently, V2 highlights the effectiveness of synthetic data compared to real data collection and labelling.
Neurolabs’ Foundational Models

Neurolabs is pioneering foundational models to optimise retail automation. We create “general-purpose” models that understand any retail scene by integrating advanced datasets and state-of-the-art models. These models excel in critical Consumer Packaged Goods (CPG) tasks like identifying Stock Keeping Units (SKUs), recognising promotions, and ensuring compliance.
Our foundational models are versatile tools capable of comprehensive scene understanding in retail environments. They adapt to various retail automation needs, making them invaluable assets for retailers and CPG companies. They provide heightened visibility across the supply chain and enable stronger, data-driven insights.
The Future
As we continue to develop our technology, we focus on addressing a wide range of applications within the CPG space, including enhancing the accuracy and reliability of automated checkout processes.
We aim to create models that provide significant value to retailers and CPG companies, driving innovation and efficiency in retail automation. Neurolabs' synthetic data-powered models are set to transform the CPG space with versatile, high-performing tools for scene understanding and beyond.
Want to find out more about our technology? Read our complete technical study now.
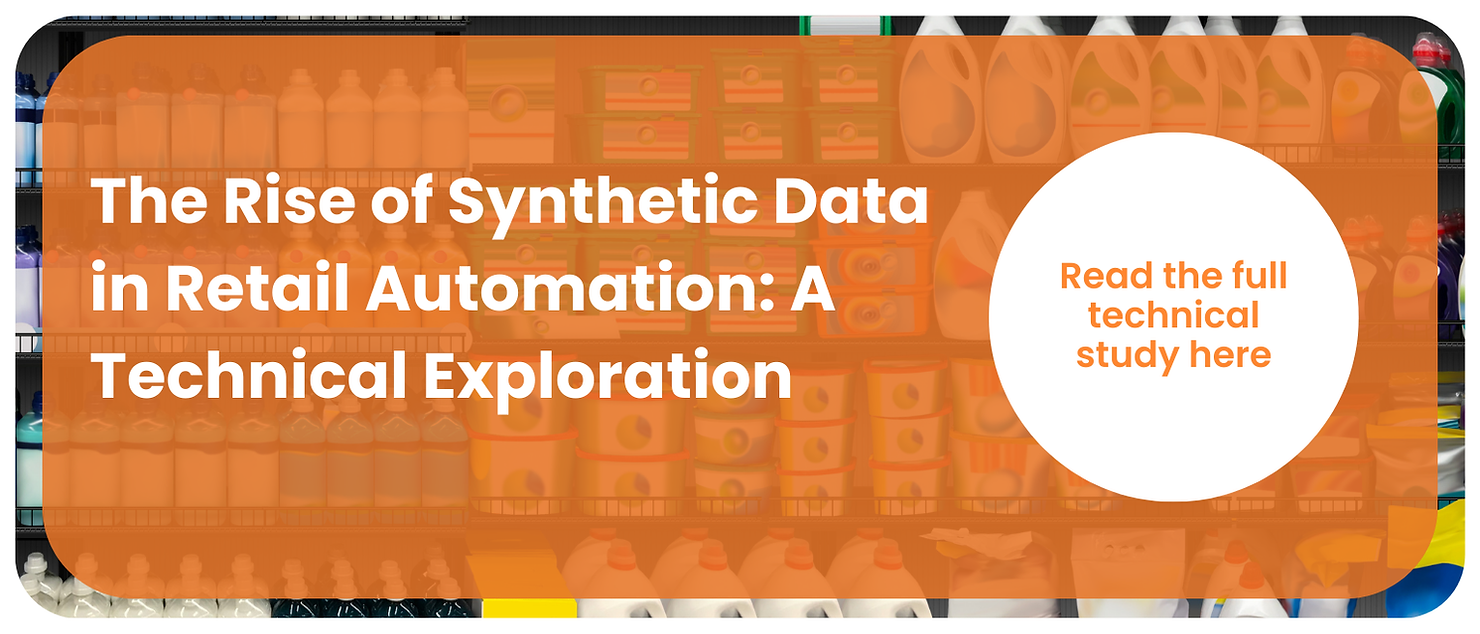