In the dynamic landscape of retail automation, data is the driving force behind innovation and efficiency. As retailers and CPGs strive to meet consumers' ever-growing demands, the need for accurate and scalable data solutions has never been more pronounced.
However, reliance on real-world data presents numerous challenges, such as scalability issues and time-consuming data acquisition and annotation processes.
These factors often render traditional IR solutions unreliable. The inability to efficiently scale data collection and annotation means that insights derived from these solutions can be incomplete or outdated, limiting their effectiveness.
As a result, CPGs may struggle to respond swiftly to market changes, optimise their operations, or tailor their strategies to meet consumer demands. Without robust and timely insights, maintaining a competitive edge in the fast-paced retail industry becomes increasingly difficult.
Therefore, innovative data generation and analysis approaches are crucial for developing more reliable and scalable IR solutions that can provide CPGs with the actionable intelligence they need to stay ahead in a competitive industry.
In response to these challenges, synthetic data has emerged as a game-changing alternative. Neurolabs, at the forefront of technological innovation, has recognised the transformative potential of synthetic data in revolutionising retail automation.
Through groundbreaking research and development, Neurolabs has pioneered innovative approaches to synthetic data management, reshaping the CPG industry and empowering businesses to thrive in an increasingly competitive market.
In this article, we explore the growing significance of synthetic data and Neurolabs' pioneering role in shaping the future of retail automation.
The Challenges of Real Data in Retail Automation
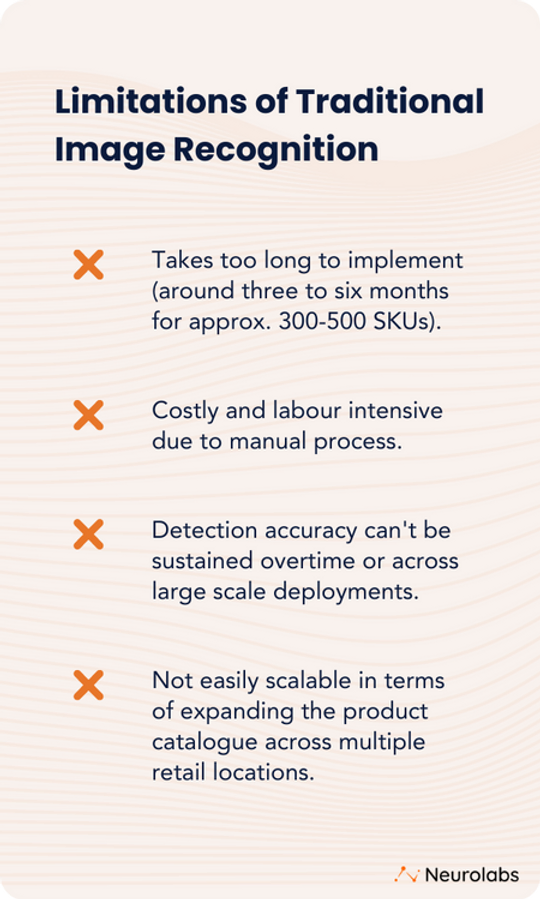
While real-world data is helpful for training computer vision models, it presents a myriad of challenges in the context of retail automation.
The quickly changing nature of retail environments, where products and packaging can change frequently, makes it difficult to maintain up-to-date datasets. This rapid turnover requires constant data collection and re-annotation, which is time-consuming and resource-intensive.
One major obstacle is the challenge of fine-grained classification. In retail, products often differ by subtle attributes such as slight variations in packaging design, size, or labelling. Distinguishing between these minute differences with IR technology requires highly detailed and precise annotations. Manually labelling such fine-grained data is laborious and prone to human error, which can compromise the accuracy of the resulting machine-learning models.
Additionally, creating realistic scene data for training purposes is another significant hurdle. Real-world data must accurately represent the complex and varied environments found in retail settings. This includes different lighting conditions, shelf arrangements, and occlusions where products partially obscure one another.
Capturing and annotating these diverse scenarios in real-world settings is incredibly challenging and often results in incomplete or biased datasets.
Overall, collecting and annotating real data is costly and time-consuming, with a higher risk of human error, potentially leading to inaccurate insights for CPGs.
Understanding Synthetic Data: The Benefits
Synthetic data, a simulated alternative to real-world data, is a cornerstone for training machine learning models and conducting simulations.
It is a meticulously crafted dataset that mimics real-world scenarios but is generated computationally rather than collected from physical sources.
Synthetic data is crucial in training foundational machine learning models, serving as the backbone for most AI applications. Unlike real data, synthetic data offers several advantages, making it an increasingly critical component of data-driven solutions.
Here are some of the key ways that Neurolabs uses synthetic data to improve retail automation processes for CPG brands:
Scalability

One key advantage of synthetic data is its scalability. Unlike real data, which may be limited in quantity and scope, synthetic data can easily be generated in vast quantities. This scalability allows for creating diverse and comprehensive datasets that capture various scenarios and variations, which is essential for robust model training.
Speed
Speed is another significant benefit of synthetic data. Manually acquiring and labelling real-world data can be time-consuming and labour-intensive. It involves visiting physical locations, capturing images, and annotating them, which can take weeks or months to complete. In contrast, synthetic data generation can be automated and accelerated, creating millions of annotated images in a fraction of the time it would take to collect and label real data.
Accuracy & Control
Synthetic data offers greater control and flexibility in the data generation process, leading to higher accuracy in model training. By creating synthetic scenarios, researchers can deliberately introduce edge cases and challenging scenarios that may be rare or difficult to encounter in the real world. This enables more robust model training and prepares AI systems for real-world deployment.
No Annotations Needed
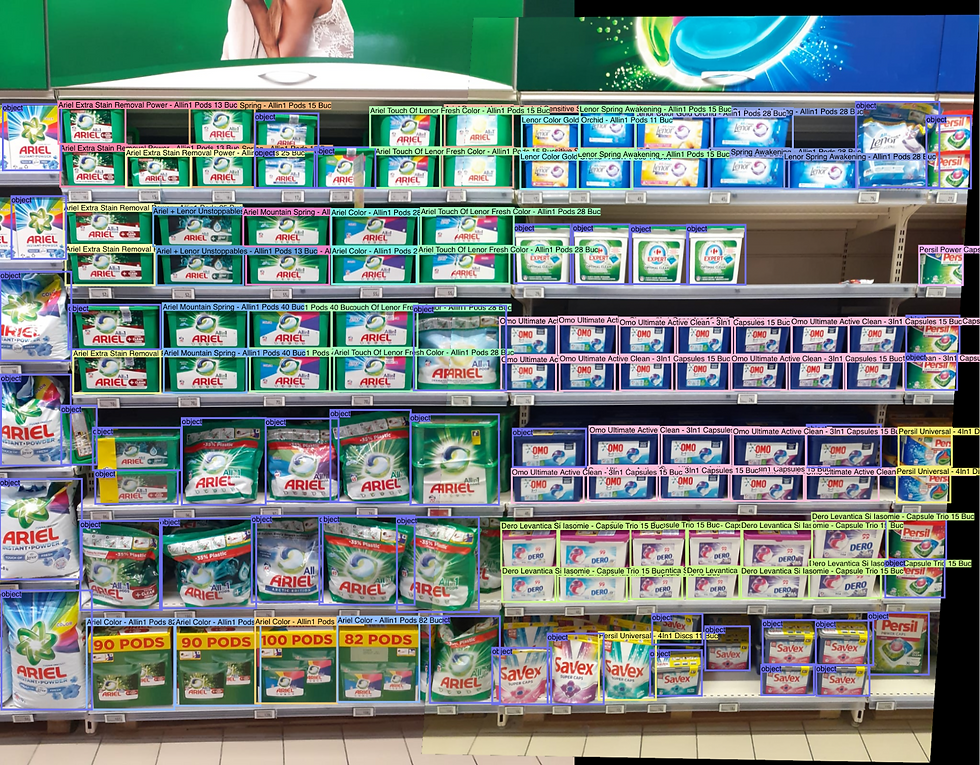
Synthetic data allows for the acquisition of annotations that would be extremely challenging or impossible to obtain in the real world. For example, annotations such as segmentation maps, depth maps, or object orientations can be generated effortlessly as part of the synthetic data generation process. This eliminates manual annotation, reduces costs, and speeds up the data preparation pipeline.
At Neurolabs, we leverage advanced computational techniques and generative models to harness the power of synthetic data, paving the way for groundbreaking innovations in retail automation and beyond.
Neurolabs' Approach to Synthetic Data Management
Neurolabs has revolutionised synthetic data management with our cutting-edge computer vision solution, ZIA (Zero Image Annotations). This innovative approach addresses the common problem of data scarcity, particularly in rapidly changing retail environments where products and packaging are frequently updated.
ZIA leverages synthetic data and advanced computer vision techniques to create comprehensive and up-to-date product catalogues for Consumer Packaged Goods (CPG) brands.
Our team demonstrating how to onboard new SKUs using the ZIA Capture app.
One of ZIA's standout features is its ability to handle fine-grained product classification—distinguishing between items that differ by minute details, which is particularly difficult with traditional data annotation methods. By automating the data generation process, ZIA ensures that CPG brands can access detailed and accurately annotated datasets, enabling more precise and effective retail execution strategies.
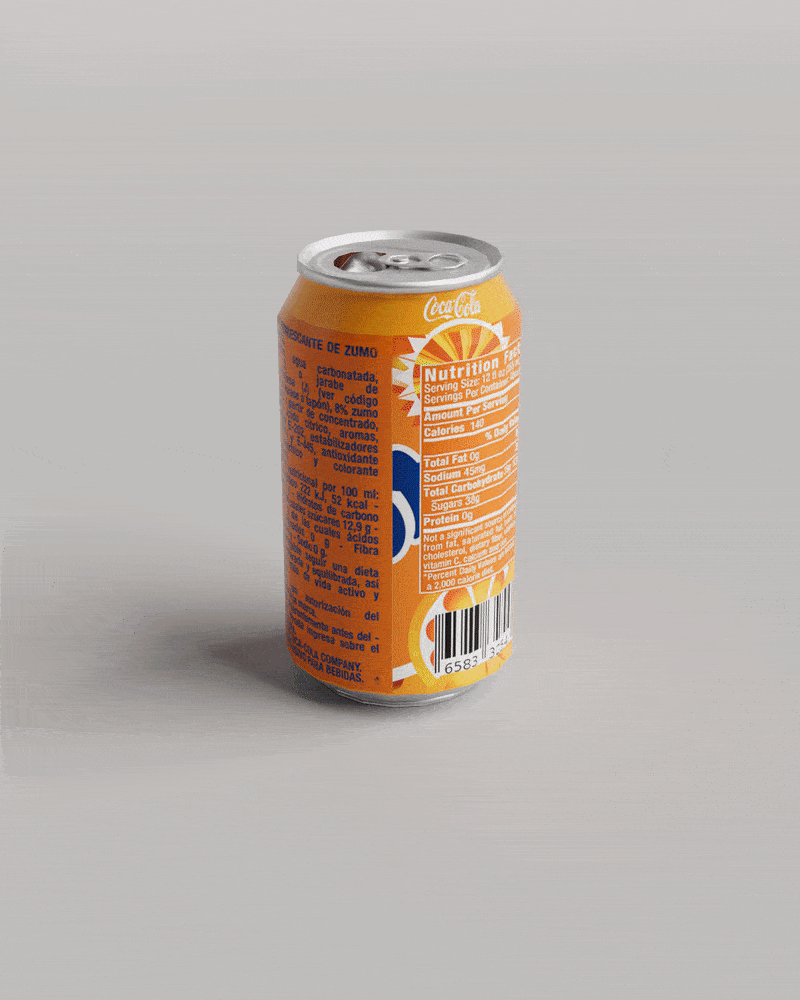
Another key component of Neurolabs' approach is the ZIA Capture app, which streamlines the onboarding process by allowing users to generate high-quality 3D models directly on the device. This feature is crucial for creating photorealistic scenes and detailed product representations, which are then used to generate synthetic data. This accelerates the data collection process and enhances the quality and diversity of the datasets, making them more robust and reliable.
Neurolabs' technology stands out from competitors due to its proactive nature and efficiency. ZIA and ZIA Capture automate the creation and organisation of product catalogues, reducing the time and effort required for manual data collection and annotation.
This automation enables CPG brands to stay agile and responsive to market dynamics, maintaining an up-to-date and accurate representation of their product offerings. By leveraging synthetic data, Neurolabs provides a scalable, accurate, and efficient solution that addresses the challenges of traditional data management in the retail sector.
The Evolution of Neurolabs’ Synthetic Data Modeling
Neurolabs has made significant strides in synthetic data modelling, evolving from SOTA vision transformer V1 to V2, a state-of-the-art transformer model.
Extending this advanced model and adapting our training strategies, we have maximised performance using synthetic data. This involves meticulous hyperparameter tweaking and employing sophisticated data augmentation techniques to bridge the gap between synthetic and real data, ensuring our models are robust and reliable.
The bulk of our innovation lies in our approach to synthetic data generation. We have developed a novel method for procedurally synthesising dense retail environments at scale. This method generates extensive variations in scene elements such as shelf structures, product assortments, and room layouts. This procedural generation allows us to create complex, annotation-rich synthetic datasets tailored for solving various computer vision tasks.
By training a state-of-the-art vision transformer model on these datasets, we have demonstrated that synthetic data alone can avoid the tedious process of real
data curation while achieving performance comparable to models trained on significantly larger mixed datasets, which predominantly consist of real images.
Remarkably, in certain environments, our synthetic-only model even outperforms those trained on mixed data, highlighting the effectiveness and potential of our synthetic data generation methods.
Datasets
Our datasets, such as NLB200k, Nuke1.0, and Nuke2.0, are the cornerstone of our intellectual property and play a crucial role in enhancing model performance. They offer a range of functions, including:
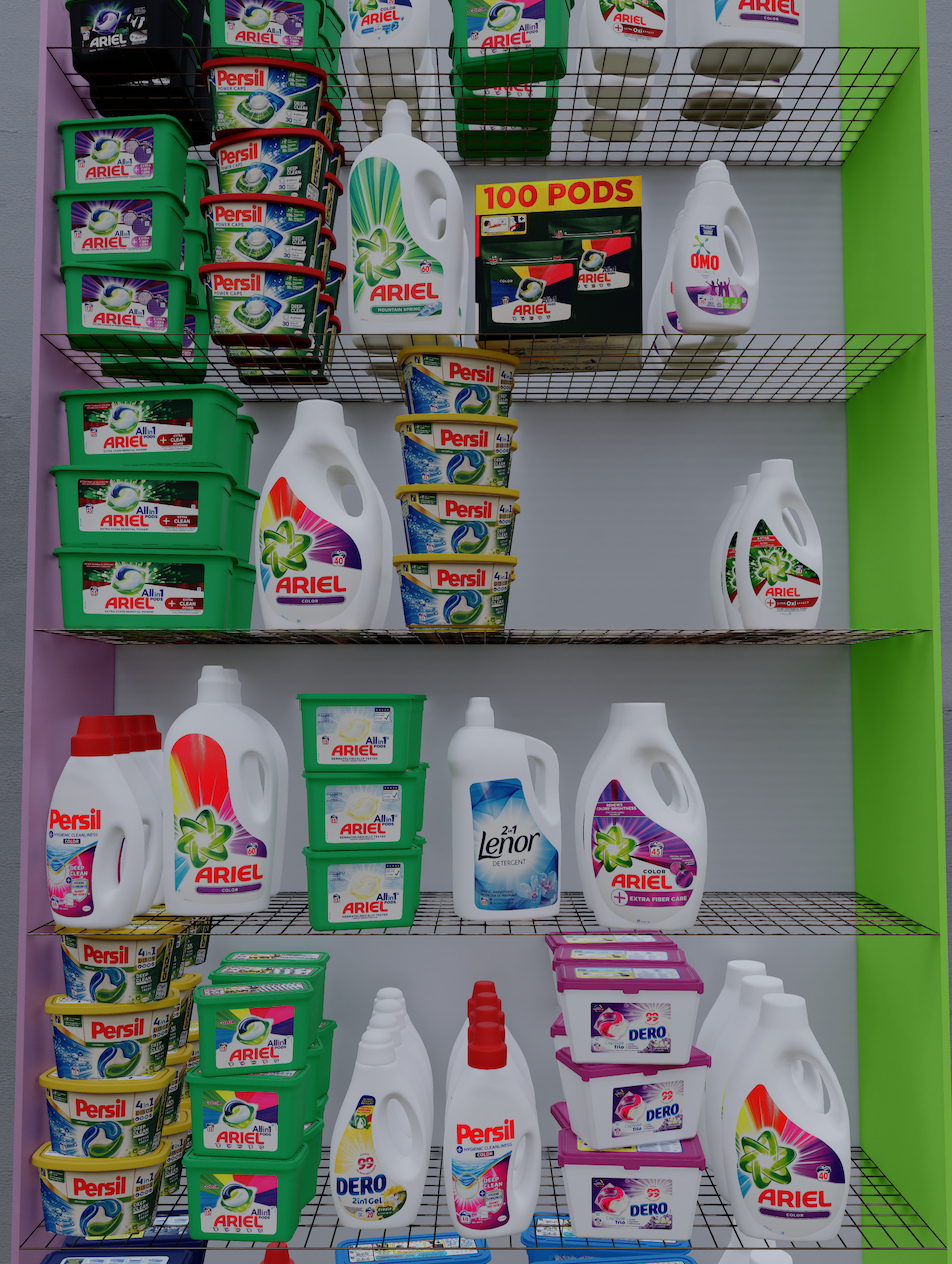
NLB200k (Synthetic Only): This dataset comprises procedurally generated retail environments, offering extensive variations in scene elements such as shelf structures, product assortments, and room layouts. It enables our models to achieve high performance using synthetic data alone, thus avoiding the tedious process of real data curation and annotation.
Nuke1.0 and Nuke2.0 (Real + Synthetic): These datasets predominantly feature real images, supplemented with synthetic data to enhance their diversity and realism. They are designed to train models that perform exceptionally well in real-world retail scenarios, facilitating tasks like fine-grained classification and detailed scene understanding.
These datasets are meticulously crafted to ensure diversity and realism, enabling our models to perform exceptionally well in various retail scenarios. These datasets' diversity and high fidelity are instrumental in training models capable of fine-grained classification and detailed scene understanding.

Models
The transition from vision transformer V1 to V2 marks a significant advancement in our modelling capabilities. Our unique approach involves tailoring them to handle larger and more complex datasets. This involves a foundational understanding of the models and aligning their evolution with the need to process and analyse extensive synthetic datasets effectively.
When comparing V1 and V2, the latter exhibits improved training times, enhanced performance, and additional features that make it superior. For instance, the size of datasets processed by V2 is substantially larger, demonstrating its capability to handle vast amounts of data efficiently.
To put this into perspective, gathering and manually labelling an equivalently sized real dataset would take several months and incur significant costs, highlighting the efficiency and cost-effectiveness of synthetic data.
Neurolabs’ Foundational Models
Neurolabs is pioneering the development of foundational models to revolutionise retail automation. Our strategy integrates the power of our advanced datasets and state-of-the-art models to create “general purpose” models that can understand any retail scene.
These foundational models are designed to excel in various critical tasks within the Consumer Packaged Goods (CPG) space, such as identifying Stock Keeping Units (SKUs), recognising promotional activities, and ensuring compliance.
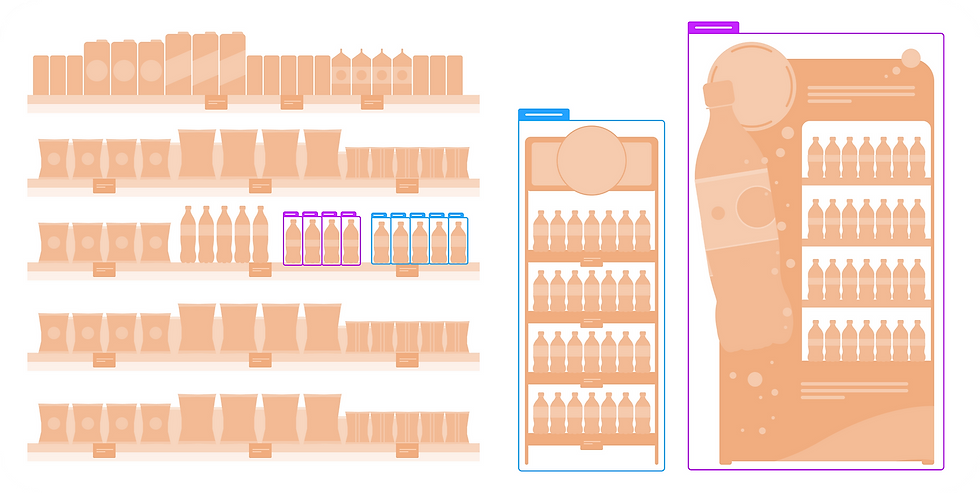
Our vision for foundational models extends to creating versatile tools capable of comprehensive scene understanding in retail environments. These models are not limited to specific tasks but are built to interpret a wide range of retail scenarios, offering unparalleled flexibility and efficiency.
This broad applicability ensures that our models can adapt to various retail automation needs, making them invaluable assets for retailers and CPG companies. Our technology gives brands heightened visibility across the entire supply chain, allowing for stronger, data-driven insights and actions.
As we continue to research and develop our technology, we are focused on addressing a wide range of applications within the CPG space, including modern challenges such as enhancing the accuracy and reliability of automated checkout processes.
We aim to develop foundational models that provide significant value to retailers and CPG companies, driving innovation and efficiency in retail automation. Our synthetic data-powered models are set to transform the CPG space by providing versatile, high-performing tools for scene understanding and beyond.
Implications for Retail Automation
The advancements in synthetic data modelling techniques have profound implications for retail automation. By leveraging V2 and our comprehensive synthetic datasets, we can create highly accurate and scalable solutions for retail automation tasks such as shelf analysis, robot picking, and automated checkouts.
These advanced models enable precise product recognition and scene understanding, facilitating efficient and effective retail operations.
Join The Future of Retail Automation with Neurolabs
In conclusion, Neurolabs' progress in creating cutting-edge synthetic data modelling techniques showcases our commitment to improving retail automation across the CPG industry.
By combining innovative data generation methods with advanced modelling strategies, we are driving the future of retail automation, offering efficient and cost-effective solutions. As we continue to refine and expand our synthetic data capabilities, the potential for further innovation and efficiency in retail automation remains vast.
If you’re interested in learning more or exploring how Neurolabs technology can improve your retail operations, contact us to book a demo today!
At Neurolabs, we are revolutionising in-store retail performance with our advanced image recognition technology, ZIA. Our cutting-edge technology enables retailers, field marketing agencies and CPG brands to optimise store execution, enhance the customer experience, and boost revenue as we build the most comprehensive 3D asset library for product recognition in the CPG industry.
Comments