In this article, we will discuss the drawbacks of legacy Image Recognition (IR), where they stem from, and the potential of synthetic data to equip retail for a new era of shelf auditing and automation.
The Root of the Problem: Manual Processes
The New Generation of Image Recognition
Market Advantage for the Taking
The Limitations of Legacy IR
When Image Recognition was first introduced into retail, it came with great promises of value across the board — more data, clearer analytics, faster insights, and automation instead of manual efforts. Years later, the industry has adopted a more sober perspective, the complexities and dynamic state of retail having taken their toll on the dream of easy and accurate in-shelf analytics.
Before going into how we can move away from legacy IR, let’s take a look at how it fell short of the retail industry’s demands and expectations.
Retail Field Execution Companies (RFEC in short) typically state the following three pain points when dealing with legacy IR:
- Slow deployment speed
- Low accuracy
- Lack of scalability
It can take months to introduce an Image Recognition model to a new catalogue of SKUs for it to recognise, with the resulting analytics rarely operating with more than 95% accuracy — a wide-enough error margin to prevent mass adoption of Image Recognition in retail.
To make matters worse, as product catalogues exist in a constant state of flux (being prone to frequent label changes, re-brands, and seasonal promotions), the long time frame to adopt even a single new SKU creates a constant uphill battle for RFEC companies — when timely and up-to-date analytics are the most critical, it can take days to adopt a changed SKU into a legacy IR system.
Lastly, unlike many other technologies, legacy IR does not profit from economies of scale, meaning that RFECs are left with high, fixed costs for each new retail location and product category they expand to. This makes legacy IR’s adoption daunting at best and commercially unviable at worst.
Below are some direct quotes we hear time and time again from our clients regarding legacy IR and its most common pain points.

The Root of the Problem: Manual Processes
Visual detection algorithms require contextual information to learn and improve over time — with legacy IR, this additional data is provided through the manual annotation of real-world images. However, both the accumulation of real-world images and their annotation are time-consuming and expensive — when speaking of days or weeks to adopt a set of new SKUs, this is where these delays are caused, with no available shortcuts for the process.
These workflows aren’t only slow, but also entirely reactionary — a new SKU can only be photographed and annotated once it has already hit in-store shelves. There are no results or analytical data from day one.
Lastly, the prowess of legacy visual detection models relies on workers’ ability to correctly identify and interpret every piece of visual information forwarded to the AI. When dealing with pictures of cluttered shelves, half-hidden products, and bad lighting conditions, this becomes a difficult and time-consuming exercise, even for the most qualified of annotators.
Although legacy IR providers such as Trax and Parallel Dots have attempted to meet client expectations and market demands, they have not been able to consistently do so due to their reliance on real data.
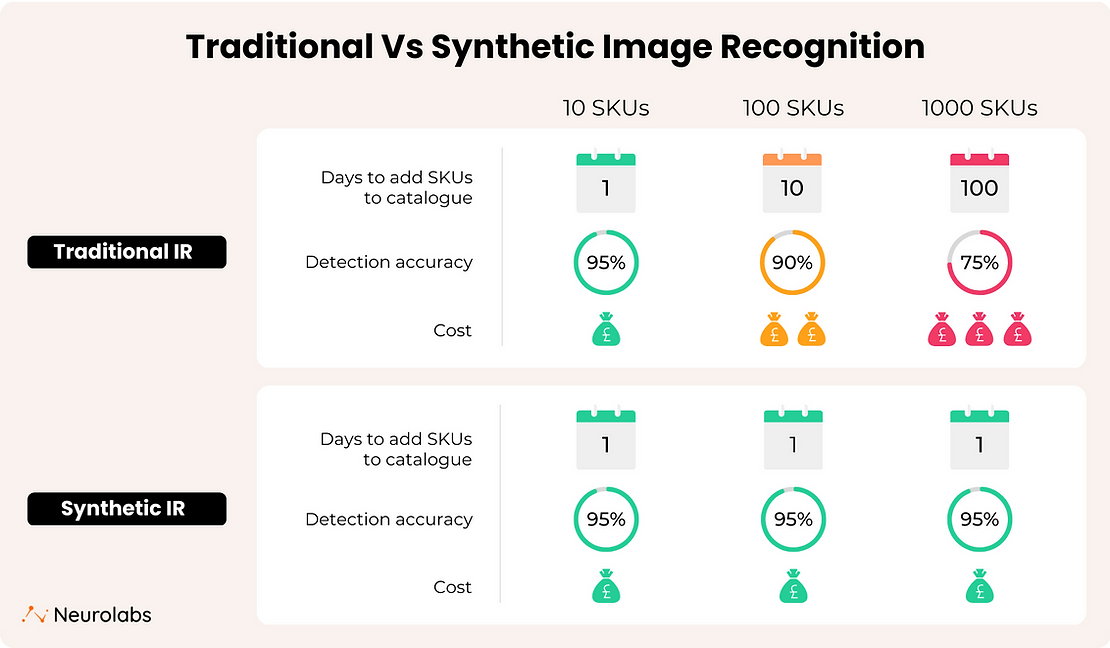
The New Generation of Image Recognition
We’ve recently seen the advent of a new wave of Image Recognition, systems powered by a combination of synthetic data and modern computer vision, with the ability to circumnavigate the need for real data altogether. This leap in automation technology has led to large shifts in focus across a variety of industries and applications, including retail.
Rather than relying on a collection of manually annotated real-world images, these systems turn SKUs into digital 3D models for Image Recognition algorithms to learn from and reference. The needed contextual data is automatically provided through a variety of virtual environments these digital SKUs are then placed in — specifically, which products are which and where they are positioned within the 3D space. In short, synthetic data replaces the need for real data which is largely inaccurate, quick to expire, expensive and time-consuming to collect.
Next to outperforming legacy IR solutions in every aspect, staying native to a digital environment also unlocks entirely new possibilities for RFECs and their clients alike. For instance, for the first time ever, it is possible to train models for changes in SKU labelling and branding before these changes affect real-world shelves, allowing for proactive initiatives and zero-delay analytics.
Below is a short overview of how things happen in the new paradigm:

You can also explore our case study with IPP to take a closer look at a real-world deployment of this technology.

Market Advantage for the Taking
When legacy IR became the norm in retail, it provided a valuable stepping stone for modern shelf auditing — however, though proving automation tech’s potential, it never made true on its promises to fulfil it. Now, with the adoption of synthetic data, we are able to usher in an entirely new era of Image Recognition, making good on old promises and once again expanding our understanding of what is possible for retail.
As with any large tech shift, some providers may be slow to embrace the new generation of Image Recognition — but CPGs and retailers will readily move on from real data systems to synthetic alternatives once the full weight of upcoming recessions hits their customers and in-shelf performance. Sped up by these economic developments, and following the trends across various other industries, it won’t take long for synthetic data and computer vision to establish themselves as go-to solutions within the retail space.
At Neurolabs, we are proud to be at the forefront of this tech revolution, busily providing RFECs with the new generation of Image Recognition. Our web-based platform is the first comprehensive destination for RFECs to take advantage of new-wave IR within the shelf-auditing space and our clients are ambitious market-leaders whom we’ve seen gain significant and sustainable advantages over their competition. As experts for computer vision and synthetic data at heart, we look forward to continue showcasing the technology’s potential and being the No.1 partner for the pioneers of the retail space.
To explore the benefits of new-wave Image Recognition first hand, book a demo here. You can also explore our blog to learn more about our technology and its possible applications.
Retailers worldwide lose a mind-blowing $634 Billion annually due to the cost of poor inventory management; with 5% of all sales lost due to Out-Of-Stocks alone.
Neurolabs helps optimise in-store retail execution for supermarkets and CPG brands using a powerful combination of Computer Vision and Synthetic Data, improving customer experience and increasing revenue. Our goal is to build the largest 3D asset repository for the CPG space.