Using virtual products to solve real-world problems

Customers Come First
The international ITAB Group is a leading supplier of high-quality retail store solutions such as cash desks, self-checkout, and customer guidance systems. They design, manufacture, deliver, and install retail solutions from end to end using the most innovative technologies available.
Their primary aim is to offer each customer a first-class service and their highest priority is always customer satisfaction. This is no easy feat when you consider the rapidly changing demands of the modern consumer combined with constantly increasing competition amongst Fast Moving Consumer Goods (FMCG) brands.
To ensure that they can continue to maintain such stellar levels of service and keep their retail customers happy at scale, they have implemented many automated solutions. One such system involves the use of image recognition technology to automate visual-based tasks for retailers. Specifically, they have experimented with Computer Vision (CV) to streamline the processes involved in shelf monitoring and inventory management in supermarkets and retail stores.
As part of their experiments, they explored a new type of CV known as Synthetic Computer Vision (SCV) and sought to put the new technology’s capabilities to the test.

Virtual Reality
Synthetic Computer Vision (SCV) is an innovative, AI-powered approach to image recognition that enables the user to automate the detection of real world objects such as Fast Moving Consumer Goods.
In order to detect an object in the real world, the technology is trained using a virtual replica of that object. Images and videos are obtained using this virtual replica, or 3D asset, that, when combined with rendered images and videos of the 3D asset places in 3D, virtual scenes, compose the Synthetic Data. In short, the Synthetic Data is a computer generated version of a supermarket shelf with products on it.
By using Synthetic Data for CV, the technology radically reduces the cost and time traditionally required to train and deploy image recognition models. This makes the automation of visual-based tasks such as shelf monitoring in supermarkets so much simpler.
ITAB take a diligent approach when it comes to choosing the right system for improving in-store experience for their customers. They are strategic when it comes to choosing a solution that will withstand the test of time and easily scale across many locations so exploring the use of SCV was a no-brainer.
ITAB wanted to test the capabilities of Neurolabs’ SCV platform ZIA to see whether it could improve the performance of their existing CV models. These models would be used to automate in-store shelf monitoring. If successful, they aimed to switch to using fully Synthetic Datasets for future CV applications due to the time and cost saving provided by the use of Synthetic Data versus real data alone.
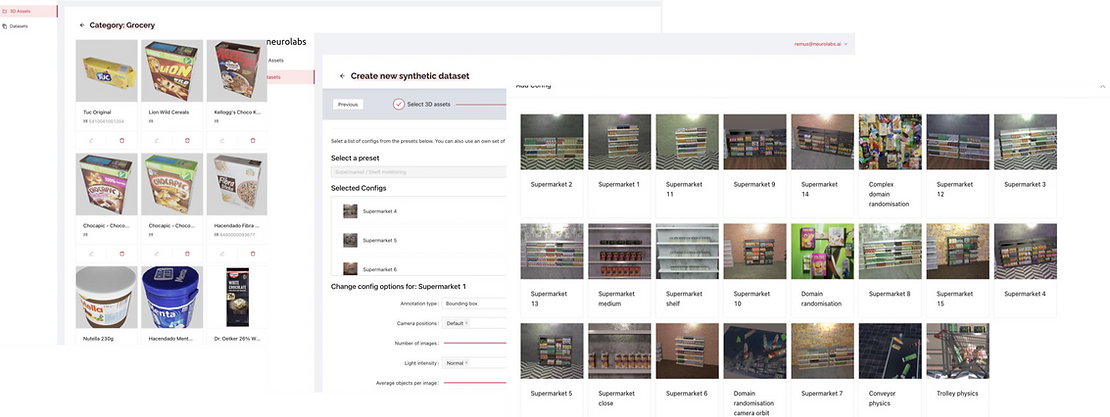
Proof of Concept
In order to prove that SCV would perform in a real world retail environment, ITAB set up a lab environment, consisting of multiple products on a supermarket shelf, to test it.
The purpose of the project was twofold:
- To evaluate the effectiveness of the detection of Out-Of-Stock products using SCV
- To investigate the efficiency and scalability of using Synthetic Data versus real data to train CV models to detect products on supermarket shelves
Focusing on a sample of 50 different Stock-Keeping Units (SKUs) across multiple categories, they set out to prove whether Synthetic Data could improve their workflows and, ultimately, benefit the end customer.

The SCV Process
1. Digital Twins
To get started, ITAB needed high quality training data. Instead of the traditional approach to CV of capturing and labelling vast amounts of real images, SCV simplifies the initial data requirement to just one virtual recreation of the object that is being detected. In this case, they needed virtual recreations of their supermarket products.
Neurolabs ZIA created virtual versions of each of their SKUs using six images of each product. These images were used to build a virtual, 3D model for each SKU. The end result were 3D assets that were ready to be uploaded to ZIA as the input for the Synthetic Dataset generation stage. The platform automatically labelled each of the products in the process.
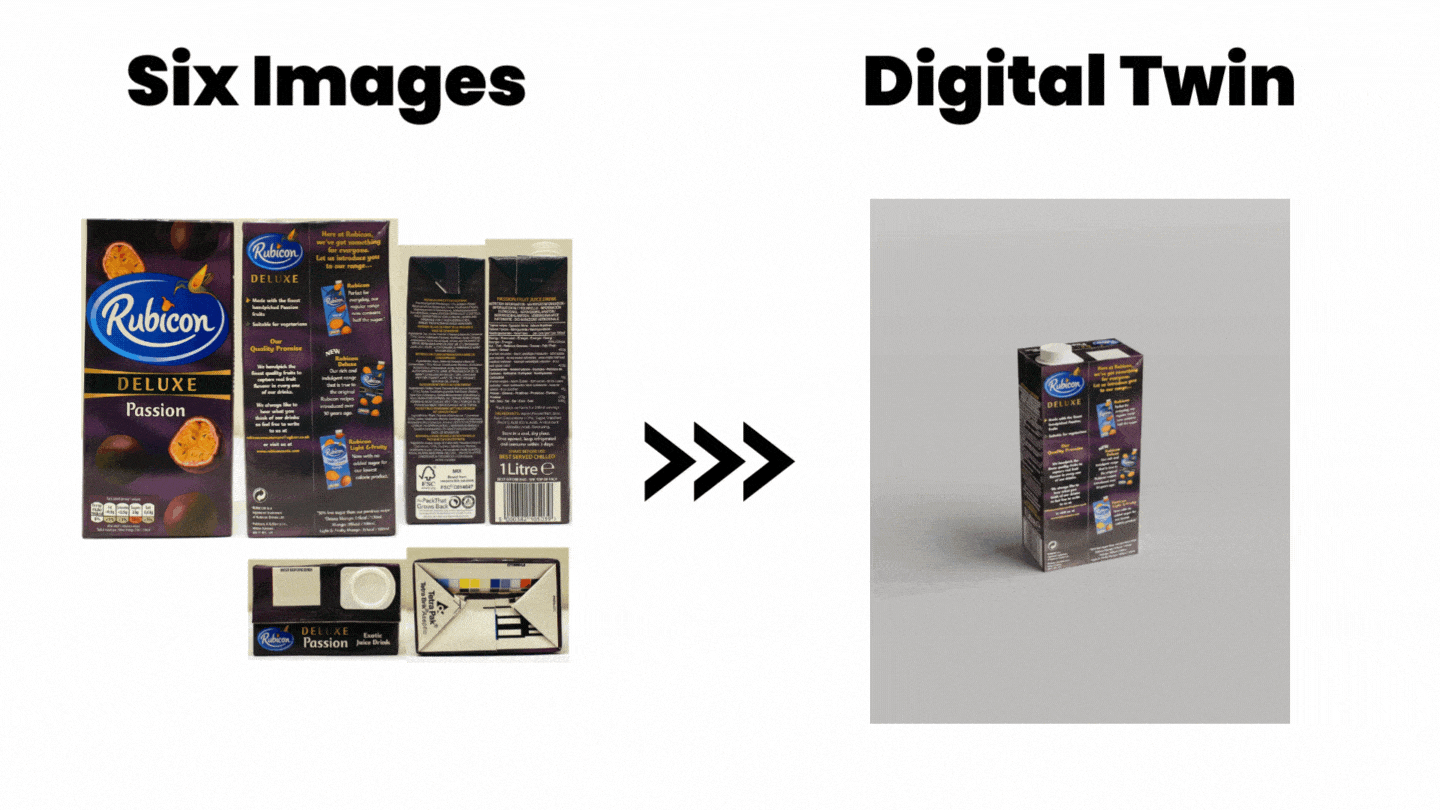
2. Synthetic Datasets
Once the 3D asset was uploaded to Neurolabs platform, ZIA, various 3D virtual scenes were automatically generated and Synthetic Datasets, in the form of 2D images, were created, to represent the multiple ways that the product can appear in real life. These datasets are used as examples for the CV algorithms to learn how the product may look like in varying, different environments i.e. to train a CV model.
For ITABs use case, the datasets contained variations of their virtual products in very different scenes to help train their CV model to carry out shelf monitoring in very different, real-world retail environments.
There are three specialised techniques that Neurolabs offers to generate Synthetic Data on the platform:
- Using Domain Randomization,
- Using Pre-Existing Scenes,
- Using ITABs own scenes sourced from AutoCAD models of their customers’ stores.
ITAB tested all the three methods to see which worked best for them.
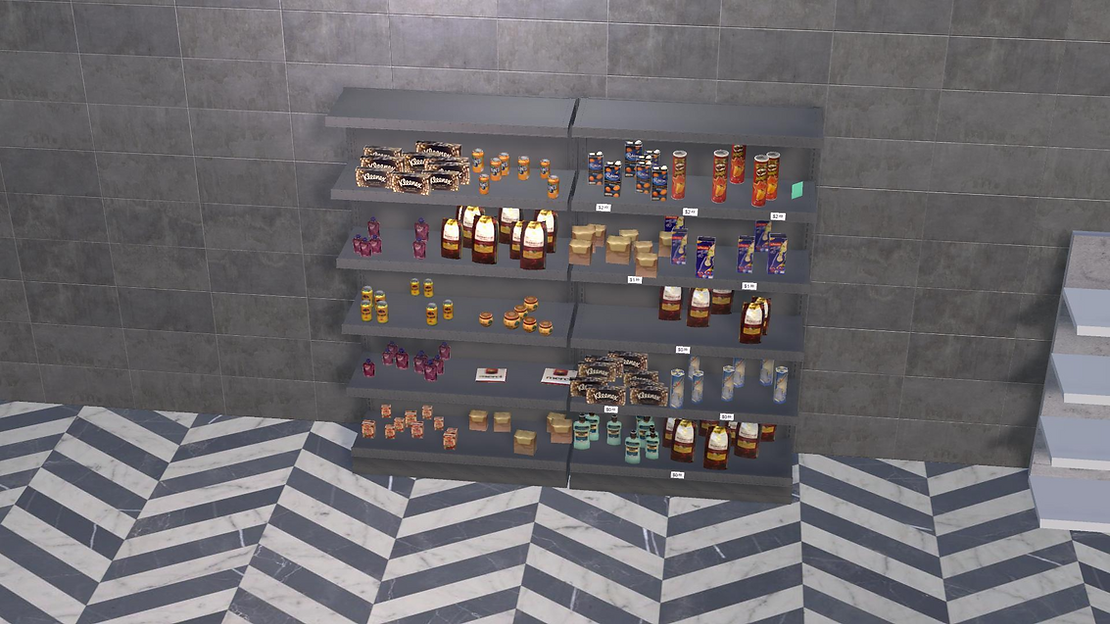
3. Model Training
Once the datasets were ready, ITAB used them to train a CV model on the Neurolabs platform, ZIA. They were able to rapidly deploy that same model to detect the supermarket products in the lab environment that they had created.
Deploying advanced image recognition technology with ZIA is as simple as making an API call.

Tried and True
Once ITAB had deployed their SCV model and tested its ability to carry out automated shelf monitoring, they compared the performance of the model with one trained using real data and they were very pleasantly surprised by the results.
They were also very impressed with the ease and speed of the SCV process and how seamless the Synthetic Dataset came together.

They see it as a promising technology and are pushing for it to be deployed it in a real-world retail environment as soon as possible.
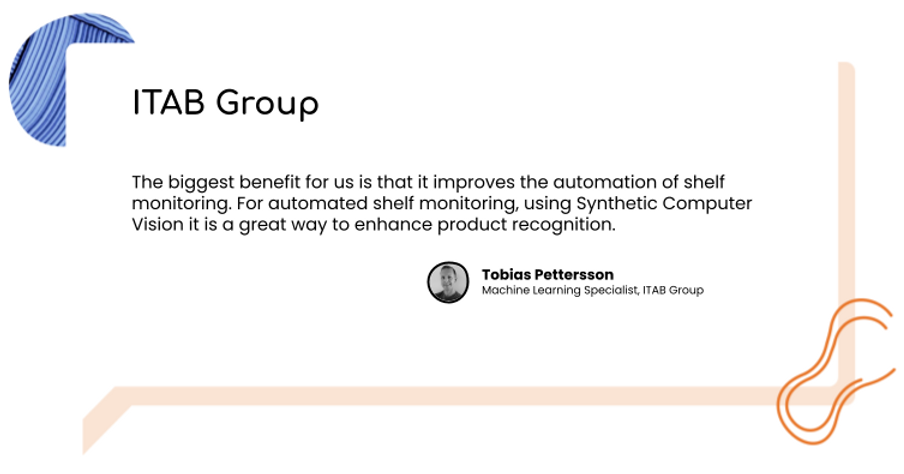
By using Synthetic Computer Vision to automate in-store shelf monitoring, ITAB was able to build a CV solution that excelled where conventional solutions are limited in many ways:
- Speed: A real-world deployment can be implemented in less than one week.
- Scale: Access to image recognition datasets for over 100,000 SKUs through the ZIA platform.
- Quality: Achieve 95% accuracy for SKU-level product recognition from day 1 and increase to above 98% for specific categories..
The most innovative retail solution providers like ITAB, who embrace state-of-the-art image technology like SCV, are ensuring a future of many very happy customers for years and years to come.
At Neurolabs, we are revolutionising in-store retail performance with our advanced image recognition technology, ZIA. Our cutting-edge technology enables retailers, field marketing agencies and CPG brands to optimise store execution, enhance the customer experience, and boost revenue as we build the most comprehensive 3D asset library for product recognition in the CPG industry.